Govern AI risk with the NIST RMF: policies, procedures, and compliance
Part 2 of a series on the National Institute of Standards and Technology's Artificial Intelligence Risk Management Framework.
Everyone loves the word “governance.”
Same with “AI.”
So in this post I’ll combine the two by looking at the first sub-part of the first function of the NIST Artificial Intelligence Risk Management Framework (RMF), called GOVERN. This article builds on the first part of this series, where I looked at framing AI risk with the RMF. Check that out first if you want the full picture.
Zooming out, you’ll see that it is at the center of an ongoing cycle described by the RMF:
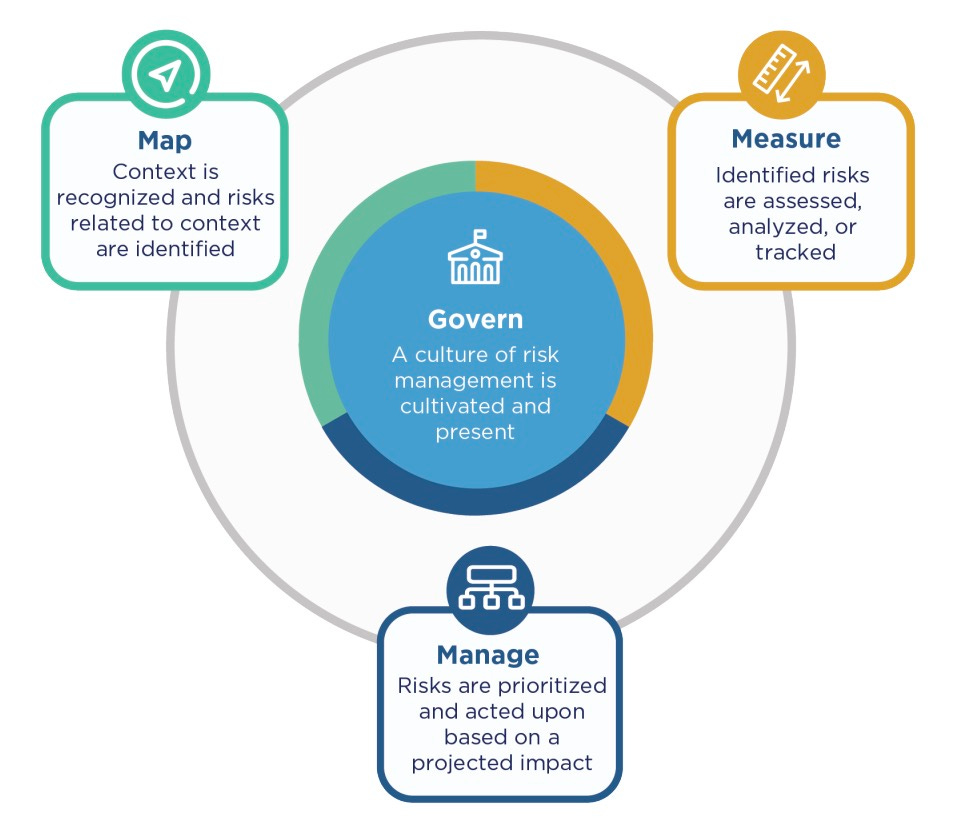
The GOVERN function comprises six numbered parts, each having their own sub-bullets. After attempting to get them all in a single post, I realized that fully exploring them required substantial analysis. So in this post I am just going to focus on the first sub-bullet. I’ll tackle subsequent ones individually or in groups in later articles.
1. Policies, processes, and procedures
1.1 Legal and regulatory requirements understood and met
This is a very broad requirement that itself could require several full time employees to cover. To provide some examples, though, these could require ensuring compliance with the
Various vague and threatening Federal Trade Commission (FTC) statements
Health Insurance Portability and Accountability Act (HIPAA)
Federal Communications Commission (FCC) Privacy and Data Protection obligations
European Union (EU) General Data Protection Regulation (GDPR)
California Consumer Privacy Act (CCPA) and Privacy Rights Act (CPRA)
Other U.S. state data privacy laws
1.2 Trustworthy AI integrated
For a description of what “trustworthy” means, please review my first post.
1.3 Risk management practices driven by risk tolerance
This seems high-level and obvious, but it’s important to explore the second-level implications here. From what I understand, this is saying that just because you have a documented risk tolerance doesn’t mean you are done.
You actually need to use that tolerance to drive your risk management practices.
I have seen organizations with documented security policies where those drafting the policies had no idea how to enforce the risk tolerance, had not consulted those doing the implementation, and those expected to implement the policies ignored the written requirements.
This is equally undesirable in AI-related situations. So make sure you have a plan to put AI risk management practices into action, rather than just rubber-stamping a boilerplate policy and calling it a day.
1.4 Risk management implemented transparently
Transparency is easy to talk about but hard to implement. Organizations managing complex systems of all kind are often intentionally opaque about their risk management practices and tolerances due to bureaucratic infighting and efforts to avoid accountability.
This makes life extremely difficult for those on the “sharp end,” who need to juggle vague, competing, and sometimes contradictory demands. And they react by sometimes doing strange things.
If you sometimes don’t like how people react to these circumstances, you really aren’t going to like what AI systems with no common sense do.
So communicating risk management parameters in explicit, quantitative, and machine-readable terms is vital. Even when it is humans reading the guidance, they will thank you for being clear.
1.5 Continuous monitoring in place
As with cybersecurity issues, the level of risk monitoring required is closely tied to the organization’s risk tolerance and management posture.
Autonomous vehicles might require monitoring every millisecond by an independent system to ensure they are operating within specified parameters. With an AI-powered meme generator, though, it might be perfectly reasonable to just wait for customer support cases to roll in to let you know when it is broken or malfunctioning.
The potential impact of an adverse event is what should drive the frequency and granularity of monitoring here.
1.6 AI system asset inventory
Knowing exactly which AI tools are operating in your network or have access to your data is a key consideration. Unfortunately, considering that few organizations have an accurate asset inventory of their existing IT infrastructure to begin with, this is probably going to be a heavy lift.
Things get especially muddy now that vendors are launching AI-driven asset discovery tools. A good first question to ask during a demo of one of these would be “show me the tool doing an asset scan identifying itself.”
If the tool can’t do this, then think hard about how accurate your asset - especially of AI tools - will be.
With that said, due to the heightened regulatory scrutiny over AI, you might want to prioritize identifying these systems first. And keeping a close watch on which ones are introduced, and how they are used.
1.7 Decommissioning procedures established
As a security-focused product manager at previous companies, I frequently accumulated odd and sundry responsibilities that had security implications but weren’t traditionally thought of as security related.
Product support and end-of-life policies was one of those responsibilities. And believe me when I say that these things are often a security nightmare!
Customers of software products generally pay little attention to which versions they are using, what the release cadence is, and what the vendor’s communicated vulnerability notification and disclosure policy is.
That all changes when there is a problem.
Here is a brief list of urgent and serious questions and complaints you can expect to field related to this topic:
“What do you mean you won’t support that version anymore? We spent six months deploying it!”
“Why isn’t there a security patch available for this extremely severe vulnerability in our out-of-support product?”
“We can’t apply that security patch for an extremely severe vulnerability because it will break our production system that patches together a hodgepodge of cutting-edge and borderline-obsolete software!”
I think the pain caused by these situations will pale in comparison to that caused by the decommissioning of AI systems.
To mitigate these, some things ask are:
“What is the vendor support policy for this model?”
“Oh, this is an open-source model…well who do we go to if the project maintainers just disappear?”
“Is it conceivable we would permanently shut down the model in production due to extreme behavior or the discovery of a severe and effectively unpatchable security issue?”
“How would we communicate with customers, partners, regulators, executives, and other stakeholders about all of the above?”
And of course, you’ll need to have detailed processes in place to handle data destruction, minimization, or transfer when you conduct a scheduled decommissioning of a system.
Think about this ahead of time. Thank me later.
Conclusion
Even the first sub-bullet of the GOVERN function is a blog post’s worth of material, so reviewing the AI RMF in its entirety looks like its going to be a huge project. Because there has been relatively little work done on the topic so far, though, I think it will be worth it.
If you disagree, by all means let me know.
Barring that, stay tuned for the next issue. I’ll continue my teardown of the remaining sub-bullets in this section, weaving in Deploy Securely’s unique cyber risk management principles throughout.
Want to manage risk during your AI-powered transformation?